일 | 월 | 화 | 수 | 목 | 금 | 토 |
---|---|---|---|---|---|---|
1 | ||||||
2 | 3 | 4 | 5 | 6 | 7 | 8 |
9 | 10 | 11 | 12 | 13 | 14 | 15 |
16 | 17 | 18 | 19 | 20 | 21 | 22 |
23 | 24 | 25 | 26 | 27 | 28 | 29 |
30 |
- Segment Anything
- cvpr 논문 리뷰
- 컴퓨터비전
- 논문 리뷰
- CVPR
- cvpr 2024
- visual language model
- Data-centric AI
- Computer Vision 논문 리뷰
- medical image
- deep learning 논문 리뷰
- Computer Vision
- Segment Anything 리뷰
- VLM
- Self-supervised learning
- Segment Anything 설명
- LLM
- Vision
- open-world
- Stable Diffusion
- Data-centric
- Multi-modal
- Prompt Tuning
- Meta AI
- 논문리뷰
- deep learning
- contrastive learning
- 자기지도학습
- 딥러닝
- Prompt란
- Today
- Total
목록논문리뷰 (5)
Study With Inha
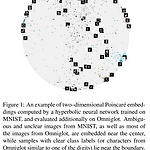
CVPR 2020, Hyperbolic Image Embeddings 논문 링크: https://arxiv.org/abs/1904.02239(https://arxiv.org/abs/1904.02239) Hyperbolic Image Embeddings Computer vision tasks such as image classification, image retrieval and few-shot learning are currently dominated by Euclidean and spherical embeddings, so that the final decisions about class belongings or the degree of similarity are made using linear hy ..
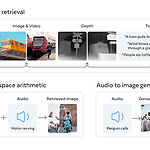
Meta AI (현 메타 에이아이, 구 페이스북), Facebook Research team (FAIR) IMAGEBIND: One Embedding Space To Bind Them All 논문 링크: https://arxiv.org/pdf/2305.05665.pdf 1. Introduction 최근 Segment Anything Model (SAM) 이라는 것을 발표한 Meta AI에서 또 다른 논문을 발표했다. 2023년 5월 9일 아카이브에 올라온 최신 논문인데, 신선한 아이디어를 제시하고 있어 리뷰할 논문으로 선정했다. 이 논문에서 제시하는 'ImageBind'는 여러가지 모달리티들의 embedding을 하나의 공통 space에 정렬함으로써 긴밀한 관계를 형성하고, 이를 통해 다양한 multi-..
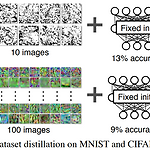
MIT CSAIL, T. Wang et al, Dataset Distillation, 2018 논문 링크: https://arxiv.org/pdf/1811.10959.pdf 1. Introduction 일반적으로 딥러닝에서는 대용량의 데이터셋으로 큰 모델을 학습시키는 것이 좋은 성능을 내고 있다. 하지만 그 경우 많은 메모리, 노동력, 시간 등등의 자원이 필요하므로 효율성 측면에서 좋은 학습 방법이라고 말하기는 어렵다. 그래서 많은 연구진들이 적은 자원으로 최대한의 효율을 낼 수 있는 방법론들에 대한 연구를 진행했고, 본 논문에서 소개하는 'Dataset Distillation (데이터셋 증류)' 도 그 중 하나라고 볼 수 있다. 데이터셋 증류란 대규모 데이터셋을 대표되는 몇 장의 합성 이미지로 압축한 ..
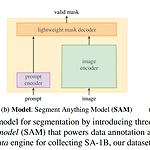
Meta AI, Segment Anything, Alexander Kirillov et al.논문 링크: https://ai.facebook.com/research/publications/segment-anything/ 1. Introduction2023년 4월 5일에 Meta AI가 공개한 Segment Anything이라는 논문은 모든 분야에서 광범위하게 사용할 수 있는 image segmentation model 에 대해서 설명하고 있다. 앞으로 segmentation 관련 연구들의 foundation이 되는 모델이 될 수 있도록 하는 것이 목표라는 엄청난 포부로 시작된다... 이 때문에 광범위한 대용량 데이터셋 (SA-1B)을 새롭게 만들고, 이것으로 학습을 시켜 powerful generaliz..
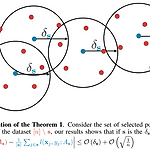
Core-set: Active Learning for Convolutional Neural Networks 논문 링크: https://arxiv.org/abs/1708.00489 Active Learning for Convolutional Neural Networks: A Core-Set Approach Convolutional neural networks (CNNs) have been successfully applied to many recognition and learning tasks using a universal recipe; training a deep model on a very large dataset of supervised examples. However, this approach i..