일 | 월 | 화 | 수 | 목 | 금 | 토 |
---|---|---|---|---|---|---|
1 | 2 | |||||
3 | 4 | 5 | 6 | 7 | 8 | 9 |
10 | 11 | 12 | 13 | 14 | 15 | 16 |
17 | 18 | 19 | 20 | 21 | 22 | 23 |
24 | 25 | 26 | 27 | 28 | 29 | 30 |
- Computer Vision 논문 리뷰
- 논문 리뷰
- Segment Anything 리뷰
- ai 최신 논문
- contrastive learning
- 논문리뷰
- Computer Vision
- CVPR
- 컴퓨터비전
- cvpr 2024
- ssl
- Meta AI
- iclr 2024
- VLM
- cvpr 논문 리뷰
- Self-supervised learning
- object detection
- 자기지도학습
- Stable Diffusion
- deep learning
- active learning
- deep learning 논문 리뷰
- Prompt란
- Data-centric AI
- Multi-modal
- Prompt Tuning
- Segment Anything
- iclr 논문 리뷰
- Data-centric
- Segment Anything 설명
- Today
- Total
목록분류 전체보기 (26)
Study With Inha
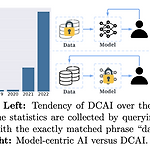
Data-centric AI: Perspectives and Challenges https://arxiv.org/pdf/2301.04819v3.pdf 앤듀르 응(Andrew Ng) 교수님께서 최근 강조하고 계시는 Data-centric AI란 무엇인지, 그리고 왜 필요한가에 대해 Data-centric AI: Perspectives and Challenges 논문을 리뷰하면서 간단하게 소개하고자 한다. 1. Data-centric AI란? 이전까지 딥러닝을 연구할 때 중요했던 것은 데이터로 '모델'을 학습하는 것이었다. 하지만 우리는 이 과정에서 '어떤 데이터'로 모델을 학습하는지에 따라서 성능이 크게 좌우된다는 것을 느껴왔다. 따라서 사람들은 학습 데이터가 단순히 '모델'을 학습하기 위한 연료 개념이 ..
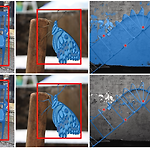
Segment Anything in High Quality, ETH Zurich 논문링크: https://arxiv.org/abs/2306.01567 Segment Anything in High QualityThe recent Segment Anything Model (SAM) represents a big leap in scaling up segmentation models, allowing for powerful zero-shot capabilities and flexible prompting. Despite being trained with 1.1 billion masks, SAM's mask prediction quality falls short inarxiv.org Introduction올해 상..
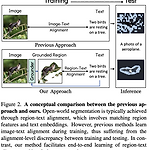
CVPR 2023 accepted paper, TCL (Text-grounded Contrastive Learning): Learning to Generate Text-grounded Mask for Open-world Semantic Segmentation from Only Image-Text Pairs 논문링크: https://arxiv.org/abs/2212.00785 Learning to Generate Text-grounded Mask for Open-world Semantic Segmentation from Only Image-Text Pairs We tackle open-world semantic segmentation, which aims at learning to segment arbit..
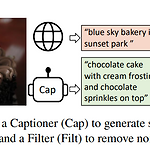
BLIP: Bootstrapping Language-Image Pre-training for Unified Vision-Language Understanding and Generation 논문 링크: https://arxiv.org/abs/2201.12086 BLIP: Bootstrapping Language-Image Pre-training for Unified Vision-Language Understanding and Generation Vision-Language Pre-training (VLP) has advanced the performance for many vision-language tasks. However, most existing pre-trained models only excel..
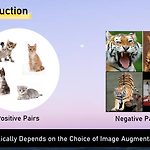
[Self-supervised Learning 개론 관련 이전 글] [Self-Supervised Learning 개론 - 1] [Self-Supervised Learning 개론 - 1] Do we need labels?; Contrastive Learning부터 Deep Generative Model까지 ⚽ GOAL 2020 ~ 2023 사이에 활발하게 이루어진 연구들의 개념을 알아본다 각 개념의 대표적인 논문들을 간단하게 소개하여 연구의 흐름을 알아본다 이를 통해서 본인 연구/개발에서 써 볼만한 insigh 2na-97.tistory.com [Self-Supervised Learning 개론 - 2] [Self-Supervised Learning 개론 - 2] Do we need labels?; C..
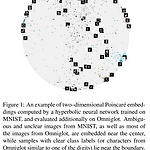
CVPR 2020, Hyperbolic Image Embeddings 논문 링크: https://arxiv.org/abs/1904.02239(https://arxiv.org/abs/1904.02239) Hyperbolic Image Embeddings Computer vision tasks such as image classification, image retrieval and few-shot learning are currently dominated by Euclidean and spherical embeddings, so that the final decisions about class belongings or the degree of similarity are made using linear hy ..
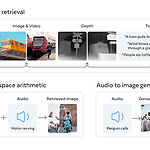
Meta AI (현 메타 에이아이, 구 페이스북), Facebook Research team (FAIR) IMAGEBIND: One Embedding Space To Bind Them All 논문 링크: https://arxiv.org/pdf/2305.05665.pdf 1. Introduction 최근 Segment Anything Model (SAM) 이라는 것을 발표한 Meta AI에서 또 다른 논문을 발표했다. 2023년 5월 9일 아카이브에 올라온 최신 논문인데, 신선한 아이디어를 제시하고 있어 리뷰할 논문으로 선정했다. 이 논문에서 제시하는 'ImageBind'는 여러가지 모달리티들의 embedding을 하나의 공통 space에 정렬함으로써 긴밀한 관계를 형성하고, 이를 통해 다양한 multi-..
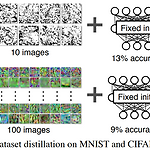
MIT CSAIL, T. Wang et al, Dataset Distillation, 2018 논문 링크: https://arxiv.org/pdf/1811.10959.pdf 1. Introduction 일반적으로 딥러닝에서는 대용량의 데이터셋으로 큰 모델을 학습시키는 것이 좋은 성능을 내고 있다. 하지만 그 경우 많은 메모리, 노동력, 시간 등등의 자원이 필요하므로 효율성 측면에서 좋은 학습 방법이라고 말하기는 어렵다. 그래서 많은 연구진들이 적은 자원으로 최대한의 효율을 낼 수 있는 방법론들에 대한 연구를 진행했고, 본 논문에서 소개하는 'Dataset Distillation (데이터셋 증류)' 도 그 중 하나라고 볼 수 있다. 데이터셋 증류란 대규모 데이터셋을 대표되는 몇 장의 합성 이미지로 압축한 ..
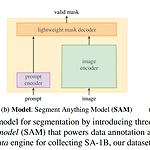
Meta AI, Segment Anything, Alexander Kirillov et al.논문 링크: https://ai.facebook.com/research/publications/segment-anything/ (SAM 후속 논문 리뷰 링크) Meta AI, SAM 2: Segment Anything in Images and Videos 논문 리뷰 및 SAM2 설명 [Paper Review] Meta AI, SAM 2: Segment Anything in Images and Videos 논문 리뷰 및 SAM2 설명Meta FAIR SAM 2: Segment Anything in Images and Videos SAM2 설명 및 논문 리뷰Paper: SAM2 Paper LinkDemo: https..
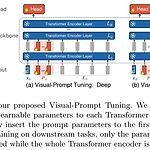
ECCV 2022, Visual Prompt Tuning, M. Jia et al. 논문 링크: https://arxiv.org/pdf/2203.12119.pdf 1. Introduction 최근 GPT 계열 모델과 같이 대규모 데이터와 대규모 모델을 활용한 딥러닝 연구가 많아졌다. 그러한 데이터의 경우 엔비디아나 구글과 같이 엄청난 컴퓨팅 파워를 가지고 있는 대기업이 아닌 일반인들은 Pretrain된 모델을 Fine-tuning하는 것도 어려운 상황에 이르렀다 :( 따라서 본 논문에서는 비전 분야에서 대규모 Transformer 모델을 효율적으로 활용하기 위한 새로운 fine-tuning 방법인 Visual Prompt Tuning (VPT)을 제안한다. 이는 기존의 fine-tuning 방법보다 더 ..
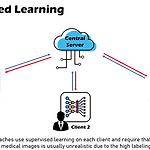
Wu et al. / Federated Contrastive Learning for Volumetric Medical Image Segmentation / MICCAI 2021 Oral Federated Contrastive Learning for Volumetric Medical Image Segmentation 1. Problem Definition 해당 논문에서는 의료 영상으로 인공지능 모델을 학습할 때 겪는 대표적인 두 가지 문제를 제시했다. 레이블(label)이 있는 데이터로 학습을 시키는 지도 학습(Supervised Learning)은 많은 분야에서 좋은 결과를 보이고 있으나, 의료 데이터의 레이블을 구하기 위해서는 의료 전문가들이 필요하며 상당한 시간을 요구하기 때문에 레이블이 있는 방대한..
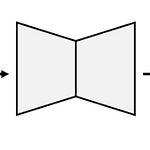
https://2na-97.tistory.com/2 [Self-Supervised Learning 개론 - 1] 링크 [Self-Supervised Learning 개론 - 1] Do we need labels?; Contrastive Learning부터 Deep Generative Model까지 ⚽ GOAL 2020 ~ 2023 사이에 활발하게 이루어진 연구들의 개념을 알아본다 각 개념의 대표적인 논문들을 간단하게 소개하여 연구의 흐름을 알아본다 이를 통해서 본인 연구/개발에서 써 볼만한 insigh 2na-97.tistory.com (1) SSL - Generative Learning 🛠️ : 입력 이미지를 넣은 후 생성된 출력 이미지에 대한 loss를 비교하는 방법 (by L1, L2 loss…) ..